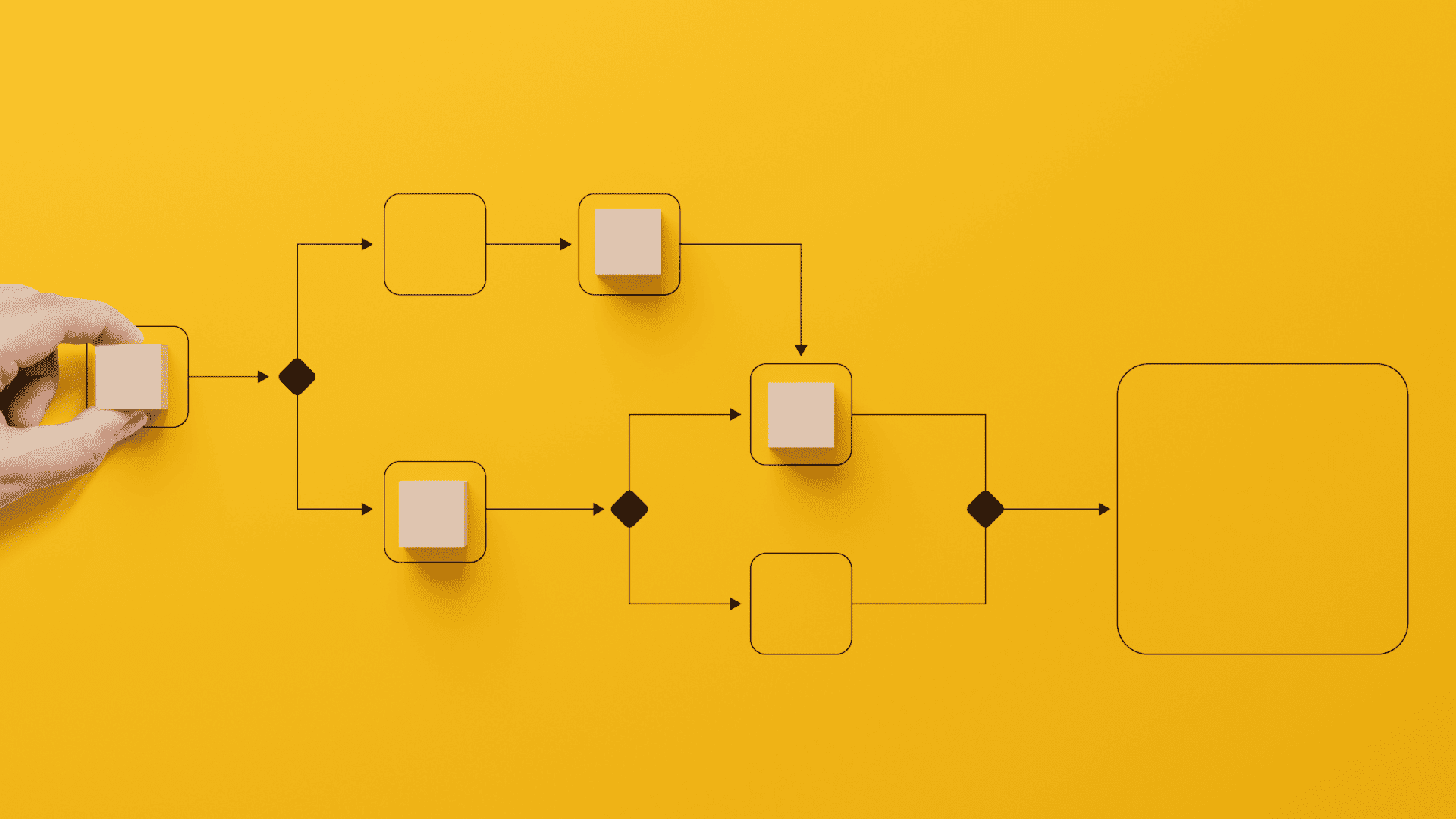
How Softwire helped Tax Systems leverage AI LLMs to transform data assessment and tax workflows
The full story
The hype and excitement around large language models (LLMs) like ChatGPT, has been enormous. The true game-changing value of LLMs emerges when businesses harness AI to perform repetitive or time-consuming tasks that require expert human judgement. Leveraging AI LLMs in this capacity enhances the efficiency of skilled professionals and business workflows.
Softwire helped us quickly test out our theories and prove that LLMs could be used to solve this very real customer challenge. And what they produced at the end was significantly more impressive than we’d expected.”
Russell Gammon
Chief Solutions Officer,
Tax Systems
Enhancing product customer experience with LLM technology
Tax Systems, a global tax and accounting software company, is the leading provider of tax compliance solutions in the UK and Ireland – used by 80% of top accountancy firms and more than 40% of FTSE 100 companies, it had identified some possible uses for LLMs.
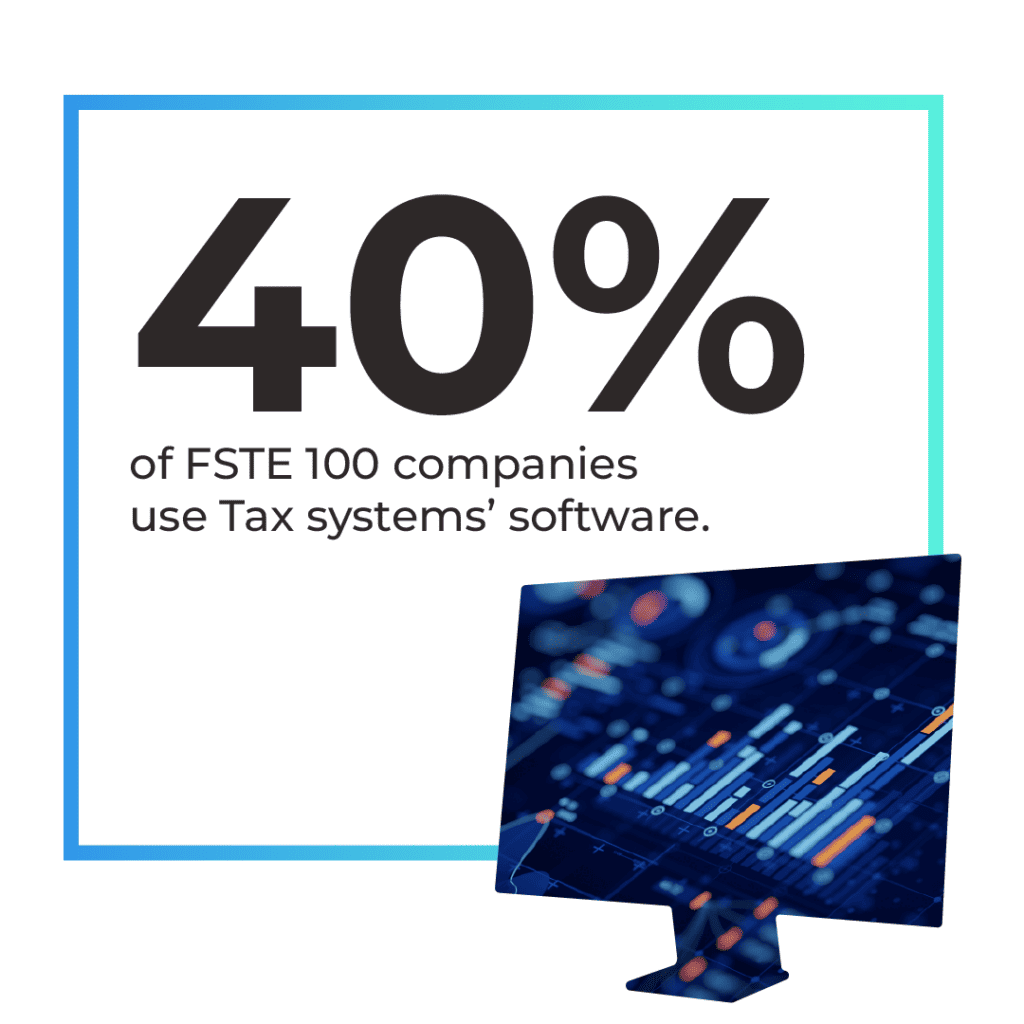
Russell Gammon, Chief Solutions Officer, explains: “Anyone who’s ever done a corporation tax computation will know how much time it takes to manually allocate the correct category to each item of revenue and expenditure, and then determine whether expenses are allowable for tax purposes.
Granted, this probably sounds like a niche pursuit. But between them, our customers do over two-hundred thousand corporation tax returns every year. When you consider that each computation takes an average of five hours to populate, much of which is manual work, it’s clear why we’ve long wanted to help our customers find a better way of doing this.
We’d explored automating these tasks. But the technology had never been capable of achieving the accuracy we needed. We were really excited to see if a large language model could change that.”
Rapid LLM innovation: Partnering with Softwire for speed and expertise
With so much innovation happening around LLMs, Tax Systems knew that speed to market would be essential. It needed a partner that would excel at working at speed, was agile in applying the technology to different use-cases and could work with scientific rigour to rapidly try multiple innovative applications, measuring the efficacy, and iterating until the end goal was reached.
Russell explains: “Softwire was one of relatively few organisations with experience in artificial intelligence and large language models. More importantly, with first-mover advantage being crucial, Softwire was able to rapidly deploy experts, who delivered the first concrete results within two weeks.”
Navigating accuracy, expertise and efficiency challenges in solution formulation
Our project team quickly understood the client’s industry and the processes where they would be looking to use AI to help.
To begin with, when preparing a company’s tax return, internal revenue and expenditure records need to be mapped to a standardised set of categories approved by HMRC. This step is normally performed by a professional tax accountant because it requires subject-matter expertise and human judgement to determine classifications.
For the first half of our solution, we needed to see if we could use OpenAI large language models to map any customer’s list of revenue and expense categories to the approved chart of accounts categories, and HMRC Detailed Profit and Loss (DPL) taxonomies. If we could crack this, it promised to eliminate thousands of hours’ manual work every year across Tax Systems’ customer base.
To get there, we needed to overcome a whole host of challenges:
Most importantly, could we achieve high enough accuracy levels to give tax professionals sufficient faith in the AI? Based on conversations with our customers, 85% accurate was determined to be the minimum trust threshold before our AI solution would be considered trustworthy, viable and useful.
Then, there was the challenge that accounting classifications required judgement. For example, subscription fees are likely income items if you’re a gym or SaaS product company but classed as expenditures if you’re an accountancy firm. Professionals rely on understanding the business’s context and their wider knowledge of the company to determine what classification to make.
The complexity and variety of input data meant that 100% accuracy wouldn’t be feasible, so how would we ensure the tax specialist using the tool knew which, out of hundreds or even thousands of entries, needed sense-checking? After all, if they had to validate every single line, this would negate the value of the system entirely.
In addition, we needed to ensure no client-sensitive information was sent to the OpenAI LLM.
Another issue here with using an OpenAI LLM was that there were limits on the rate of requests that could be sent, so we needed to use creative solutions to give users a high-quality experience in spite of the API rate limits.
Crafting a tailored solution that achieved 93% accuracy
We built and gradually refined a process capable of taking any set of input categories and mapping them to the standardised account categories. This involved working closely with Tax Systems’ corporation tax specialists to check the accuracy of the results.
Then, with careful prompt engineering and adding information around the context of the business in question, we were able to achieve accuracy levels well in excess of the 85% threshold – hitting 93% accuracy.
We carefully optimised the user experience to rapidly return the first set of classifications, while processing larger volumes in the background, allowing users to start reviewing the results without needing the whole process to be completed, thereby saving time and improving the overall experience for the tax professional.
Then, to help the user understand which entries need a human sense-check, we included a confidence score alongside each item. Any below a set limit are flagged for their attention, and they can amend them directly in the software if they need to.
Enhancing tax deduction decisions with LLM fine-tuning
Once items have been mapped to the HMRC-approved categories, the next step in the tax return process is to decide whether each item of expenditure can be deducted from the company’s profits before tax is calculated. This is traditionally done by trained professionals, who look at the wider context of the business and make assessments.
For our prototype to work, we needed to see if the LLM could correctly determine whether each item of expenditure was allowable or disallowable for tax purposes, or indeed whether it was a different category, such as the disposal of a fixed asset.
The project team initially tried the same approach as we had for mapping between charts of accounts but found this didn’t achieve the required accuracy. We quickly established that fine-tuning of the models would be required, feeding them carefully curated examples to help improve the quality of their outputs.
Fine-tuning is a technique used in machine learning to improve the performance of pre-trained models on specific tasks by training a pre-trained model with a new, relevant dataset. In this case, our team worked with tax experts to curate sample datasets that improved the model’s accuracy over a series of iterations and removed the need for examples to be supplied in the prompt.
Last, we moved on to optimising the prototype’s performance of classifying each item in a large company’s tax computation. Due to our work massively reducing the prompt sizes allowed by the fine-tuning, the prototype was able to complete this final piece of tax analysis within a few seconds
Customer-Approved: Game-changing tax prototype impresses
Now for the final test: it was time to see what Tax Systems’ customers thought. To enable it to demo what we’d created, we combined the two parts of the solution into a branded web app. The results showed that the prototype made quite an impression.
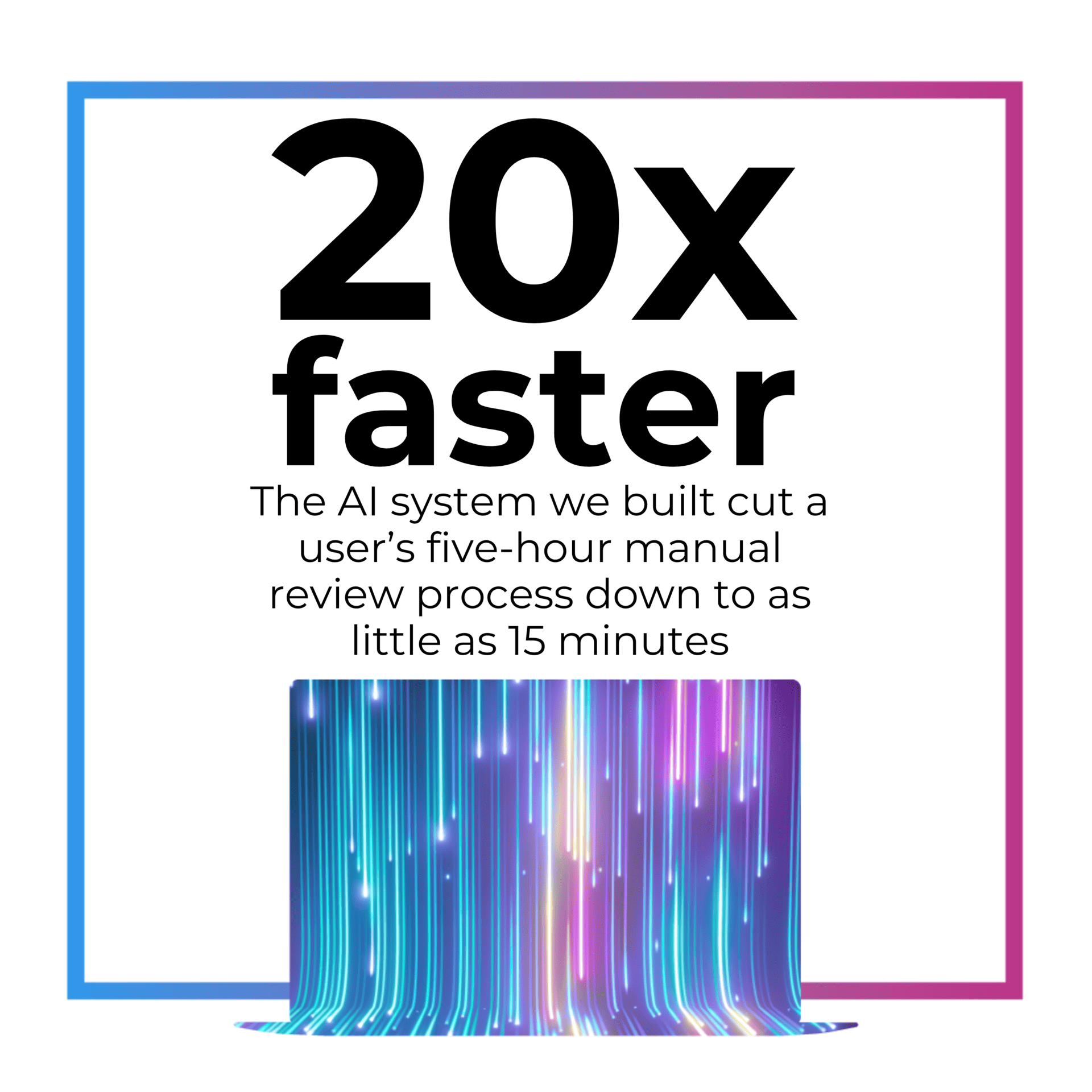
Russell picks up the story:
“The people we showed were really excited, to the point where we hadn’t even finished the demo, and some were asking us where they could sign up.
The potential to have a tax professional eliminate the need to spend four or five hours manually categorising every line item and instead take around 15 minutes to quickly check the entries the AI wasn’t sure about is genuinely game-changing. Just think how much more high-value work these skilled people could do in the time they’ll save.
Having the working, high-performance prototype that Softwire built for us was key in this regard. It meant we could demo to our customers a real system, plug their actual data into it, and show that it produced the results they’d expect – and fast.”
Having the working, high-performance prototype that Softwire built for us was key. It meant we could demo to our customers an actual system, plug their actual data into it, and show that it produced the results they’d expect – and fast.”
Russel Gammon
Chief Solutions Officer
Tax Systems
Moving Forward: Tax Systems’ new confidence in LLM-powered application development
Knowing that the technology could deliver, coupled with the level of enthusiasm from its customers, gave Tax Systems the confidence to press ahead with the development of an application it could roll out to customers. Since then, working with Softwire, a full-scale production product has been built, with the first clients expected to be using it in February 2024.
Russell concludes: “Softwire helped us quickly test out our theories and prove that LLMs could be used to solve this very real customer challenge. And what they produced at the end was significantly more impressive than we’d expected. We also got answers to a load of other questions we’d had around the use of LLMs and AI more generally, which will be useful in the future.
We’re really pleased that by working with Softwire we have built out a product ready for use by our customers.”
Need help with your own Generative AI or Digital Engineering projects? Speak to one of our team to access expert insight and unlock modern digital engineering solutions.