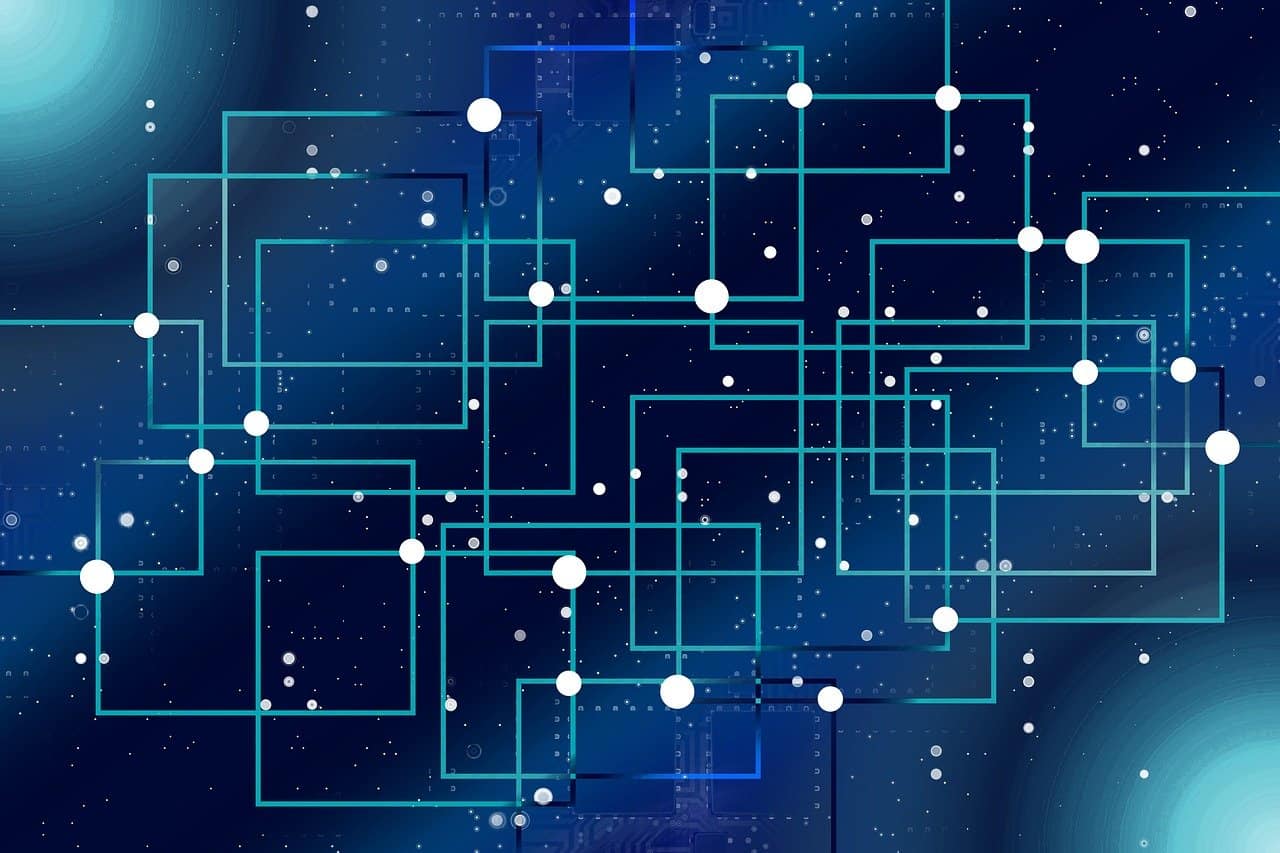
Knowing that you have data and can use it is one thing, but how do you get the best from it? Softwire’s Head of Consulting and director, Jonathon Artus, and Senior Technical Lead Rob Owen, discuss how CTOs can put data at the forefront of their digital journeys.
In this article, they share some insights about how to approach data before delving into using it for machine learning and other functions. You can also listen to the full podcast episode, which includes an accessible transcript.
What is data engineering?
Data engineering helps make data more useful and accessible for consumers of data. To do so, data engineering must source, transform and analyse data from each system.
Data engineering can be split into two halves: testing, and defining interfaces between data systems and source/ client applications.
It’s about abstracting data and presenting it in relevant business terms that can be aggregated to derive additional value and insight.
The question is, how do we arrange a business’ data in a way that makes it useful to analysts for machine learning and reporting? And can we use governance practices to make sure data storage adheres to software privacy policies?
Focussing on governance
15 years ago, governance was not a consideration. Users now expect their data and privacy to be protected. An example of positive governance is traceability, which is the ability to be able to follow the data back to its original source.
Regulators are there to protect user data, but it does present huge engineering challenges.
If somebody questions a piece of data, being able to say where it comes from is key. We’ve seen this in the handling of COVID data. For example, journalists or fact checkers will dig into numbers and find no source for the information.
Government Statistical departments are under a lot of pressure to produce figures in these scenarios. It’s important to be able to set up an internal system to capture information that can help trace back data to the source .
Importance of GDPR
GDPR, adopted by the European Parliament in April 2016, gives service or product users the right to have data removed or anonymized. However, if their data is lifted from 10 separate applications, removing or anonymising it can be very difficult — and difficult for data engineers to prove they have done it well.
Service requests and governed data engineering approaches make those challenges easier to overcome. Sensible change control processes make sure you’re adhering to regulations and any other privacy policies you may have, whilst also being able to distribute the data from your central data store.
Quality assurance
A common oversight and risk to having high quality and complete data is missing fields. This is where data isn’t present in final outputs for analytics and is difficult to retrofit.
Quality in this context lies in the accuracy, validity, completeness, and consistency of your data. By tracking data quality, a business can pinpoint potential issues harming quality and ensure that shared data is fit to be used for a given purpose.
This is particularly relevant in relational data models, where domain-specific language is designed for managing data held in relational databases, or for stream processing.
You can improve and maintain data quality by focussing on ‘quality assurance’ as part of your data governance — first, define Quality Assurance (QA) metrics and then perform regular QA audits. You can also appoint roles such as data owners, data stewards and data custodians within your organisation and establish proper processes to ensure high data quality.
Storage
The explosion in data engineering has been driven by advancements in storage — it’s faster, cheaper and is suddenly viable to collect and access in vast amounts.
Webscale companies like Netflix, which have millions of customers, are collecting and analysing data that wouldn’t have been possible to store 10 years ago. The companies are solving problems from first principles and building strategies that are trickling into the wider development ecosystem.
Consider the three S model when looking at the ideal ways of storing your data:
- Security: To maintain the robustness of your business data, you need to make sure there is a traceable source, it meets security criteria and that it’s accessible to the right people. You’ll need to protect confidential data from unauthorized in-office staff and external suppliers you work with.
- Scalability: Every business wants to grow and you need your data infrastructure to be capable of doing so, whilst being cost-effective. It’s worth looking for a storage solution that includes the ability to scale up as your business grows.
- Segregating domains: Well-segregated business domains have multiple silos for your data, helping to separate content into separate portals to set different access control for each type of content.
Learn more about how you can make the most of your data
“Data engineering is about thinking upfront about what you need to be able to do with data you have collected. Thinking about where it’s going to come from and how much of it there is lets you plan exciting things — be that machine learning, reporting or simply consuming data from web sites or other platforms.”
Jonathon Artus, Head of Consulting and director
If you’re interested in learning about our data engineering services or the work we’ve carried out, we recommend starting with our collaboration with Google DeepMind for Moorfields Eye hospital to create an AI system that helps clinicians fast-track patients with serious eye diseases. Read the case study
You can also listen to the original podcast episode with Jonathon and Rob for more information on how data engineering can help your business.